Protein engineering is important for designing proteins with particular capabilities, however navigating the complicated health panorama of protein mutations poses a major problem, making it laborious to search out optimum sequences. Zero-shot approaches, which predict mutational results with out counting on homologs or a number of sequence alignments (MSAs), cut back some dependencies however fall quick in predicting numerous protein properties. Studying-based fashions educated on deep mutational scanning (DMS) or MAVE information have been used to foretell health landscapes alone or with MSAs or language fashions. Nonetheless, these data-driven fashions typically battle when experimental information is sparse.
Microsoft Analysis AI for Science researchers launched µFormer, a deep studying framework that integrates a pre-trained protein language mannequin with specialised scoring modules to foretell protein mutational results. µFormer predicts high-order mutants, fashions epistatic interactions, and handles insertions. With reinforcement studying, µFormer effectively explores huge mutant areas to design enhanced protein variants. The mannequin predicted mutants with a 2000-fold enhance in bacterial development price, pushed by improved enzymatic exercise. µFormer’s success extends to difficult eventualities, together with multi-point mutations and its predictions have been validated by means of wet-lab experiments, highlighting its potential for optimizing protein design.
The µFormer mannequin is a deep studying strategy designed to foretell the health of mutated protein sequences. It operates in two phases: first, by pre-training a masked protein language mannequin (PLM) on a big dataset of unlabeled protein sequences, and second, by predicting health scores utilizing three scoring modules built-in into the pre-trained mannequin. These modules—residual-level, motif-level, and sequence-level—seize totally different facets of the protein sequence and mix their outputs to generate the ultimate health rating. The mannequin is educated utilizing identified health information, minimizing errors between predicted and precise scores.
Moreover, the µFormer is mixed with a reinforcement studying (RL) technique to discover the huge area of attainable mutations effectively. The protein engineering drawback on this framework is modeled as a Markov Determination Course of (MDP), with Proximal Coverage Optimization (PPO) used to optimize mutation insurance policies. Dirichlet noise is added in the course of the mutation search course of to make sure efficient exploration and keep away from native optima. Baseline comparisons have been made utilizing fashions like ESM-1v and ECNet, and so they have been evaluated on datasets reminiscent of FLIP and ProteinGym.
µFormer, a hybrid mannequin combining a self-supervised protein language mannequin with supervised scoring modules, predicts protein health scores effectively. Pre-trained on 30 million protein sequences from UniRef50 and fine-tuned with three scoring modules, µFormer outperformed ten strategies within the ProteinGym benchmark, attaining a imply Spearman correlation of 0.703. It predicts high-order mutations and epistasis, with robust correlations for multi-site mutations. In protein optimization, µFormer, paired with reinforcement studying, designed TEM-1 variants that considerably improved development, with one double mutant outperforming a identified quadruple mutant.
In conclusion, Earlier research have proven the potential of sequence-based protein language fashions in duties like enzyme operate prediction and antibody design. µFormer, a sequence-based mannequin with three scoring modules, was developed to generalize throughout numerous protein properties. It achieved state-of-the-art efficiency in health prediction duties, together with complicated mutations and epistasis. µFormer additionally demonstrated its potential to optimize enzyme exercise, significantly in predicting TEM-1 variants in opposition to cefotaxime. Regardless of its success, enhancements may be made by incorporating structural information, growing phenotype-aware fashions, and creating fashions able to dealing with longer protein sequences for higher accuracy.
Try the Paper. All credit score for this analysis goes to the researchers of this venture. Additionally, don’t overlook to comply with us on Twitter and LinkedIn. Be part of our Telegram Channel.
For those who like our work, you’ll love our publication..
Don’t Overlook to affix our 50k+ ML SubReddit
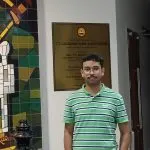
Sana Hassan, a consulting intern at Marktechpost and dual-degree pupil at IIT Madras, is captivated with making use of know-how and AI to deal with real-world challenges. With a eager curiosity in fixing sensible issues, he brings a contemporary perspective to the intersection of AI and real-life options.