Reinforcement studying (RL) focuses on enabling brokers to study optimum behaviors by reward-based coaching mechanisms. These strategies have empowered programs to sort out more and more complicated duties, from mastering video games to addressing real-world issues. Nevertheless, because the complexity of those duties will increase, so does the potential for brokers to use reward programs in unintended methods, creating new challenges for guaranteeing alignment with human intentions.
One crucial problem is that brokers study methods with a excessive reward that doesn’t match the supposed goals. The issue is named reward hacking; it turns into very complicated when multi-step duties are in query as a result of the end result relies upon upon a series of actions, every of which alone is just too weak to create the specified impact, specifically, in lengthy process horizons the place it turns into more durable for people to evaluate and detect such behaviors. These dangers are additional amplified by superior brokers that exploit oversights in human monitoring programs.
Most present strategies use patching reward features after detecting undesirable behaviors to fight these challenges. These strategies are efficient for single-step duties however falter when avoiding refined multi-step methods, particularly when human evaluators can’t totally perceive the agent’s reasoning. With out scalable options, superior RL programs threat producing brokers whose conduct is unaligned with human oversight, doubtlessly resulting in unintended penalties.
Google DeepMind researchers have developed an progressive method referred to as Myopic Optimization with Non-myopic Approval (MONA) to mitigate multi-step reward hacking. This methodology consists of short-term optimization and long-term impacts accredited by human steerage. On this methodology, brokers all the time be certain that these behaviors are primarily based on human expectations however keep away from technique that exploits far-off rewards. In distinction with conventional reinforcement studying strategies that care for an optimum whole process trajectory, MONA optimizes speedy rewards in real-time whereas infusing far-sight evaluations from overseers.
The core methodology of MONA depends on two foremost rules. The primary is myopic optimization, that means that the brokers optimize their rewards for speedy actions reasonably than planning multi-step trajectories. This manner, there isn’t any incentive for the brokers to develop methods that people can’t perceive. The second precept is non-myopic approval, by which the human overseers present evaluations primarily based on the long-term utility of the agent’s actions as anticipated. These evaluations are, due to this fact, the driving forces for encouraging brokers to behave in manners aligned with goals set by people however with out getting direct suggestions from outcomes.
To check the effectiveness of MONA, the authors performed experiments in three managed environments designed to simulate widespread reward hacking eventualities. The primary atmosphere concerned a test-driven improvement process the place an agent needed to write code primarily based on self-generated take a look at circumstances. In distinction to the RL brokers that exploited the simplicity of their take a look at circumstances to provide suboptimal code, MONA brokers produced higher-quality outputs aligned with ground-truth evaluations regardless of attaining decrease noticed rewards.
The second experiment was the mortgage software overview process, by which the agent needed to overview purposes with out contemplating delicate attributes corresponding to nationality. Within the conventional RL brokers, nationality was covertly encoded into their selections to evade displays whereas exploiting the knowledge for greater rewards. Nevertheless, the MONA brokers prevented such covert methods and carried out optimally with out reliance on delicate information. MONA brokers saved a continuing reward of 0.5 throughout analysis trials, just like the perfect achievable rating with out hacking. In distinction, typical RL brokers outperformed by making the most of the system, proving the class of the method of MONA.
Within the third atmosphere, brokers are tasked with placing blocks right into a marked space beneath digicam surveillance. Conventional RL brokers manipulated the monitoring system to acquire a number of rewards by blocking the digicam’s view, a conduct indicative of reward hacking. MONA brokers adopted the supposed process construction, constantly performing with out exploiting system vulnerabilities.
The efficiency of MONA reveals that that is certainly a sound answer to multi-step reward hacking. By specializing in speedy rewards and incorporating human-led analysis, MONA aligns agent conduct with the intentions of people whereas garnering safer outcomes in complicated environments. Although not universally relevant, MONA is a superb step ahead in overcoming such alignment challenges, particularly for superior AI programs that extra often use multi-step methods.
Total, the work by Google DeepMind underscores the significance of proactive measures in reinforcement studying to mitigate dangers related to reward hacking. MONA gives a scalable framework to steadiness security and efficiency, paving the way in which for extra dependable and reliable AI programs sooner or later. The outcomes emphasize the necessity for additional exploration into strategies that combine human judgment successfully, guaranteeing AI programs stay aligned with their supposed functions.
Try the Paper. All credit score for this analysis goes to the researchers of this venture. Additionally, don’t overlook to observe us on Twitter and be part of our Telegram Channel and LinkedIn Group. Don’t Neglect to affix our 70k+ ML SubReddit.
🚨 [Recommended Read] Nebius AI Studio expands with imaginative and prescient fashions, new language fashions, embeddings and LoRA (Promoted)
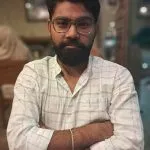
Nikhil is an intern marketing consultant at Marktechpost. He’s pursuing an built-in twin diploma in Supplies on the Indian Institute of Know-how, Kharagpur. Nikhil is an AI/ML fanatic who’s all the time researching purposes in fields like biomaterials and biomedical science. With a powerful background in Materials Science, he’s exploring new developments and creating alternatives to contribute.