Language fashions (LMs) have gained vital prominence in computational textual content evaluation, providing enhanced accuracy and flexibility. Nonetheless, a vital problem persists: guaranteeing the validity of measurements derived from these fashions. Researchers face the danger of misinterpreting outcomes, probably measuring unintended elements akin to incumbency as an alternative of ideology, or get together names somewhat than populism. This discrepancy between meant and precise measurements can result in considerably flawed conclusions, undermining the credibility of analysis outcomes.
The basic query of measurement validity looms massive within the subject of computational social science. Regardless of the rising sophistication of language fashions, issues in regards to the hole between the ambitions of those instruments and the validity of their outputs stay. This concern has been a longstanding focus of computational social scientists, who’ve constantly warned in regards to the challenges related to validity in textual content evaluation strategies. The necessity to handle this hole has grow to be more and more pressing as language fashions proceed to evolve and develop their functions throughout numerous domains of analysis.
This examine by researchers from Communication Science, Vrije Universiteit Amsterdam and Division of Politics, IR and Philosophy, Royal Holloway College of London addresses the vital concern of measurement validity in supervised machine studying for social science duties, notably specializing in how biases in fine-tuning information impression validity. The researchers goal to bridge the hole in social science literature by empirically investigating three key analysis questions: the extent of bias impression on validity, the robustness of various machine studying approaches in opposition to these biases, and the potential of significant directions for language fashions to cut back bias and enhance validity.
The examine attracts inspiration from the pure language processing (NLP) equity literature, which means that language fashions like BERT or GPT might reproduce spurious patterns from their coaching information somewhat than really understanding the ideas they’re meant to measure. The researchers undertake a group-based definition of bias, contemplating a mannequin biased if it performs unequally throughout social teams. This method is especially related for social science analysis, the place advanced ideas usually have to be measured throughout numerous social teams utilizing real-world coaching information that’s not often completely consultant.
To deal with these challenges, the paper proposes and investigates instruction-based fashions as a possible answer. These fashions obtain express, verbalized directions for his or her duties along with fine-tuning information. The researchers theorize that this method may assist fashions study duties extra robustly and scale back reliance on spurious group-specific language patterns from the fine-tuning information, thereby probably enhancing measurement validity throughout totally different social teams.
The proposed examine addresses measurement validity in supervised machine studying for social science duties, specializing in group-based biases in coaching information. Drawing from Adcock and Collier’s (2001) framework, the researchers emphasize robustness in opposition to group-specific patterns as essential for validity. They spotlight how customary machine studying fashions can grow to be “stochastic parrots,” reproducing biases from coaching information with out really understanding ideas. To mitigate this, the examine proposes investigating instruction-based fashions that obtain express, verbalized job directions alongside fine-tuning information. This method goals to create a stronger hyperlink between the scoring course of and the systematized idea, probably decreasing measurement error and enhancing validity throughout numerous social teams.
The proposed examine investigates the robustness of various supervised machine studying approaches in opposition to biases in fine-tuning information, specializing in three important classifier sorts: logistic regression, BERT-base (DeBERTa-v3-base), and BERT-NLI (instruction-based). The examine design includes coaching these fashions on 4 datasets throughout 9 kinds of teams, evaluating efficiency below biased and random coaching circumstances.
Key facets of the methodology embrace:
1. Coaching fashions on texts sampled from just one group (biased situation) and randomly throughout all teams (random situation).
2. Testing on a consultant held-out check set to measure the “bias penalty” – the efficiency distinction between biased and random circumstances.
3. Utilizing 500 texts with balanced lessons for coaching to get rid of class imbalance as an intervening variable.
4. Conducting a number of coaching runs throughout six random seeds to cut back the affect of randomness.
5. Using binomial mixed-effects regression to investigate classification errors, contemplating classifier kind and whether or not check texts come from the identical group as coaching information.
6. Testing the impression of significant directions by evaluating BERT-NLI efficiency with each significant and meaningless directions.
This complete method goals to offer insights into the extent of bias impression on validity, the robustness of various classifiers in opposition to biases, and the potential of significant directions to cut back bias and enhance validity in supervised machine studying for social science duties.
This examine investigates the impression of group-based biases in machine studying coaching information on measurement validity throughout numerous classifiers, datasets, and social teams. The researchers discovered that each one classifier sorts study group-based biases, however the results are usually small. Logistic regression confirmed the most important efficiency drop (2.3% F1 macro) when educated on biased information, adopted by BERT-base (1.7% drop), whereas BERT-NLI demonstrated the smallest lower (0.4% drop). Error chances on unseen teams elevated for all fashions, with BERT-NLI displaying the least enhance. The examine attributes BERT-NLI’s robustness to its algorithmic construction and talent to include job definitions as plain textual content directions, decreasing dependence on group-specific language patterns. These findings counsel that instruction-based fashions like BERT-NLI might supply improved measurement validity in supervised machine studying for social science duties.
Take a look at the Paper. All credit score for this analysis goes to the researchers of this venture. Additionally, don’t neglect to observe us on Twitter and be a part of our Telegram Channel and LinkedIn Group. In case you like our work, you’ll love our e-newsletter..
Don’t Overlook to hitch our 48k+ ML SubReddit
Discover Upcoming AI Webinars right here

Asjad is an intern marketing consultant at Marktechpost. He’s persuing B.Tech in mechanical engineering on the Indian Institute of Expertise, Kharagpur. Asjad is a Machine studying and deep studying fanatic who’s at all times researching the functions of machine studying in healthcare.
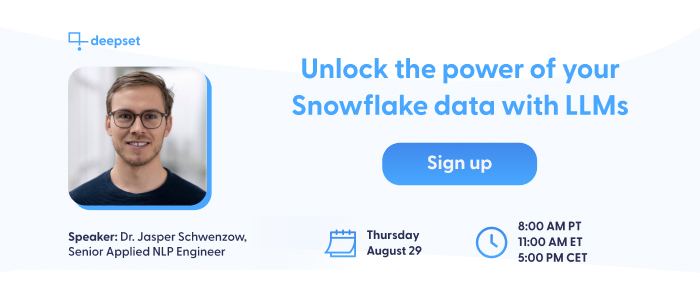