Multimodal Massive Language Fashions (MLLMs) have gained important consideration for his or her potential to deal with advanced duties involving imaginative and prescient, language, and audio integration. Nonetheless, they lack the excellent alignment past fundamental Supervised High-quality-tuning (SFT). Present state-of-the-art fashions typically bypass rigorous alignment phases, leaving essential points like truthfulness, security, and human desire alignment inadequately addressed. Present approaches goal solely particular domains comparable to hallucination discount or conversational enhancements, falling wanting enhancing the mannequin’s general efficiency and reliability. This slim focus raises questions on whether or not human desire alignment can enhance MLLMs throughout a broader spectrum of duties.
Current years have witnessed substantial progress in MLLMs, constructed upon superior LLM architectures like GPTs, LLaMA, Alpaca, Vicuna, and Mistral. These fashions have developed via end-to-end coaching approaches, tackling advanced multimodal duties involving image-text alignment, reasoning, and instruction following. A number of open-source MLLMs, together with Otter, mPLUG-Owl, LLaVA, Qwen-VL, and VITA, have emerged to deal with basic multimodal challenges. Nonetheless, alignment efforts have remained restricted. Whereas algorithms like Reality-RLHF and LLAVACRITIC have proven promise in decreasing hallucinations and enhancing conversational skills, they haven’t enhanced common capabilities. Analysis frameworks comparable to MME, MMBench, and Seed-Bench have been developed to evaluate these fashions.
Researchers from KuaiShou, CASIA, NJU, USTC, PKU, Alibaba, and Meta AI have proposed MM-RLHF, an revolutionary strategy that includes a complete dataset of 120k fine-grained, human-annotated desire comparability pairs. This dataset represents a major development when it comes to measurement, range, and annotation high quality in comparison with current sources. The tactic introduces two key improvements: a Critique-Primarily based Reward Mannequin that generates detailed critiques earlier than scoring outputs, and Dynamic Reward Scaling that optimizes pattern weights based mostly on reward alerts. It enhances each the interpretability of mannequin selections and the effectivity of the alignment course of, addressing the restrictions of conventional scalar reward mechanisms in multimodal contexts.
The MM-RLHF implementation entails a fancy knowledge preparation and filtering course of throughout three fundamental domains: picture understanding, video understanding, and multimodal security. The picture understanding element integrates knowledge from a number of sources together with LLaVA-OV, VLfeedback, and LLaVA-RLHF, with multi-turn dialogues transformed to single-turn format. This compilation leads to over 10 million dialogue samples protecting various duties from fundamental dialog to advanced reasoning. The information filtering course of makes use of predefined sampling weights categorized into three varieties: multiple-choice questions for testing reasoning and notion, long-text questions for evaluating conversational skills, and short-text questions for fundamental picture evaluation.
The analysis of MM-RLHF and MM-DPO reveals important enhancements throughout a number of dimensions when utilized to fashions like LLaVA-OV-7B, LLaVA-OV-0.5B, and InternVL-1B. Conversational skills improved by over 10%, whereas unsafe behaviors decreased by no less than 50%. The aligned fashions present higher leads to hallucination discount, mathematical reasoning, and multi-image understanding, even with out particular coaching knowledge for some duties. Nonetheless, model-specific variations are noticed, with completely different fashions requiring distinct hyperparameter settings for optimum efficiency. Additionally, high-resolution duties present restricted features on account of dataset constraints and filtering methods that don’t goal decision optimization.
On this paper, researchers launched MM-RLHF, a dataset and alignment strategy that reveals important development in MLLM growth. In contrast to earlier task-specific approaches, this technique takes a holistic strategy to enhance mannequin efficiency throughout a number of dimensions. The dataset’s wealthy annotation granularity, together with per-dimension scores and rating rationales, affords untapped potential for future growth. Future analysis instructions will deal with using this granularity via superior optimization methods, addressing high-resolution knowledge limitations, and increasing the dataset via semi-automated strategies, probably establishing a basis for extra sturdy multimodal studying frameworks.
Try the Paper and Undertaking Web page. All credit score for this analysis goes to the researchers of this venture. Additionally, be at liberty to observe us on Twitter and don’t overlook to hitch our 75k+ ML SubReddit.
🚨 Advisable Learn- LG AI Analysis Releases NEXUS: An Superior System Integrating Agent AI System and Information Compliance Requirements to Deal with Authorized Issues in AI Datasets
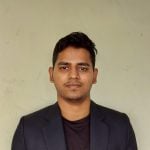
Sajjad Ansari is a closing 12 months undergraduate from IIT Kharagpur. As a Tech fanatic, he delves into the sensible functions of AI with a deal with understanding the affect of AI applied sciences and their real-world implications. He goals to articulate advanced AI ideas in a transparent and accessible method.
