Generative synthetic intelligence (AI) fashions are designed to create reasonable, high-quality knowledge, similar to photos, audio, and video, primarily based on patterns in giant datasets. These fashions can imitate complicated knowledge distributions, producing artificial content material resembling samples. One widely known class of generative fashions is the diffusion mannequin. It has succeeded in picture and video era by reversing a sequence of added noise to a pattern till a high-fidelity output is achieved. Nevertheless, diffusion fashions usually require dozens to lots of of steps to finish the sampling course of, demanding intensive computational sources and time. This problem is particularly pronounced in purposes the place fast sampling is important or the place many samples have to be generated concurrently, similar to in real-time situations or large-scale deployments.
A major limitation in diffusion fashions is the computational load of the sampling course of, which entails systematically reversing a noising sequence. Every step on this sequence is computationally costly, and the method introduces errors when discretized into time intervals. Steady-time diffusion fashions provide a technique to tackle this, as they get rid of the necessity for these intervals and thus scale back sampling errors. Nevertheless, continuous-time fashions haven’t been extensively adopted due to inherent instability throughout coaching. The instability makes it tough to coach these fashions at giant scales or with complicated datasets, which has slowed their adoption and growth in areas the place computational effectivity is vital.
Researchers have just lately developed strategies to make diffusion fashions extra environment friendly, with approaches similar to direct distillation, adversarial distillation, progressive distillation, and variational rating distillation (VSD). Every technique has proven potential in dashing up the sampling course of or enhancing pattern high quality. Nevertheless, these methods encounter sensible challenges, together with excessive computational overhead, complicated coaching setups, and limitations in scalability. As an illustration, direct distillation requires coaching from scratch, including important time and useful resource prices. Adversarial distillation introduces challenges when utilizing GAN (Generative Adversarial Community) architectures, which regularly need assistance with stability and consistency in output. Additionally, though efficient for short-step fashions, progressive distillation and VSD normally produce outcomes with restricted range or clean, much less detailed samples, particularly at excessive steerage ranges.
A analysis staff from OpenAI launched a brand new framework referred to as TrigFlow, designed to simplify, stabilize, and scale continuous-time consistency fashions (CMs) successfully. The proposed answer particularly targets the instability points in coaching continuous-time fashions and streamlines the method by incorporating enhancements in mannequin parameterization, community structure, and coaching targets. TrigFlow unifies diffusion and consistency fashions by establishing a brand new formulation that identifies and mitigates the primary causes of instability, enabling the mannequin to deal with continuous-time duties reliably. This enables the mannequin to attain high-quality sampling with minimal computational prices, even when scaled to giant datasets like ImageNet. Utilizing TrigFlow, the staff efficiently skilled a 1.5 billion-parameter mannequin with a two-step sampling course of that reached high-quality scores at decrease computational prices than present diffusion strategies.
On the core of TrigFlow is a mathematical redefinition that simplifies the likelihood movement ODE (Unusual Differential Equation) used within the sampling course of. This enchancment incorporates adaptive group normalization and an up to date goal perform that makes use of adaptive weighting. These options assist stabilize the coaching course of, permitting the mannequin to function constantly with out discretization errors that usually compromise pattern high quality. TrigFlow’s method to time-conditioning throughout the community structure reduces the reliance on complicated calculations, making it possible to scale the mannequin. The restructured coaching goal progressively anneals vital phrases within the mannequin, enabling it to succeed in stability quicker and at an unprecedented scale.
The mannequin, named “sCM” for easy, steady, and scalable Consistency Mannequin, demonstrated outcomes similar to state-of-the-art diffusion fashions. As an illustration, it achieved a Fréchet Inception Distance (FID) of two.06 on CIFAR-10, 1.48 on ImageNet 64×64, and 1.88 on ImageNet 512×512, considerably lowering the hole between the very best diffusion fashions, even when solely two sampling steps had been used. The 2-step mannequin confirmed almost a ten% FID enchancment over prior approaches requiring many extra steps, marking a considerable enhance in sampling effectivity. The TrigFlow framework represents an important development in mannequin scalability and computational effectivity.
This analysis provides a number of key takeaways, demonstrating the right way to tackle conventional diffusion fashions’ computational inefficiencies and limitations by a rigorously structured continuous-time mannequin. By implementing TrigFlow, the researchers stabilized continuous-time CMs and scaled them to bigger datasets and parameter sizes with minimal computational trade-offs.
The important thing takeaways from the analysis embody:
Stability in Steady-Time Fashions: TrigFlow introduces stability to continuous-time consistency fashions, a traditionally difficult space, enabling coaching with out frequent destabilization.
Scalability: The mannequin efficiently scales as much as 1.5 billion parameters, the most important amongst its friends for continuous-time consistency fashions, permitting its use in high-resolution knowledge era.
Environment friendly Sampling: With simply two sampling steps, the sCM mannequin reaches FID scores similar to fashions requiring intensive compute sources, attaining 2.06 on CIFAR-10, 1.48 on ImageNet 64×64, and 1.88 on ImageNet 512×512.
Computational Effectivity: Adaptive weighting and simplified time conditioning throughout the TrigFlow framework make the mannequin resource-efficient, lowering the demand for compute-intensive sampling, which can enhance the applicability of diffusion fashions in real-time and large-scale settings.

In conclusion, this examine represents a pivotal development in generative mannequin coaching, addressing stability, scalability, and sampling effectivity by the TrigFlow framework. The OpenAI staff’s TrigFlow structure and sCM mannequin successfully deal with the vital challenges of continuous-time consistency fashions, presenting a steady and scalable answer that rivals the very best diffusion fashions in efficiency and high quality whereas considerably decreasing computational necessities.
Take a look at the Paper and Particulars. All credit score for this analysis goes to the researchers of this venture. Additionally, don’t overlook to observe us on Twitter and be a part of our Telegram Channel and LinkedIn Group. In the event you like our work, you’ll love our e-newsletter.. Don’t Neglect to hitch our 55k+ ML SubReddit.
[Upcoming Live Webinar- Oct 29, 2024] The Finest Platform for Serving Wonderful-Tuned Fashions: Predibase Inference Engine (Promoted)
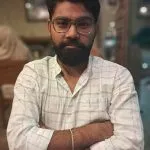
Nikhil is an intern marketing consultant at Marktechpost. He’s pursuing an built-in twin diploma in Supplies on the Indian Institute of Expertise, Kharagpur. Nikhil is an AI/ML fanatic who’s at all times researching purposes in fields like biomaterials and biomedical science. With a robust background in Materials Science, he’s exploring new developments and creating alternatives to contribute.