[ad_1]
Speculation validation is key in scientific discovery, decision-making, and data acquisition. Whether or not in biology, economics, or policymaking, researchers depend on testing hypotheses to information their conclusions. Historically, this course of entails designing experiments, accumulating knowledge, and analyzing outcomes to find out the validity of a speculation. Nevertheless, the quantity of generated hypotheses has elevated dramatically with the arrival of LLMs. Whereas these AI-driven hypotheses supply novel insights, their plausibility varies extensively, making guide validation impractical. Thus, automation in speculation validation has grow to be a necessary problem in guaranteeing that solely scientifically rigorous hypotheses information future analysis.
The principle problem in speculation validation is that many real-world hypotheses are summary and never immediately measurable. As an example, stating {that a} particular gene causes a illness is just too broad and must be translated into testable implications. The rise of LLMs has exacerbated this challenge, as these fashions generate hypotheses at an unprecedented scale, lots of which can be inaccurate or deceptive. Current validation strategies wrestle to maintain tempo, making it tough to find out which hypotheses are value additional investigation. Additionally, statistical rigor is commonly compromised, resulting in false verifications that may misdirect analysis and coverage efforts.
Conventional strategies of speculation validation embrace statistical testing frameworks resembling p-value-based speculation testing and Fisher’s mixed check. Nevertheless, these approaches depend on human intervention to design falsification experiments and interpret outcomes. Some automated approaches exist, however they typically lack mechanisms for controlling Sort-I errors (false positives) and guaranteeing that conclusions are statistically dependable. Many AI-driven validation instruments don’t systematically problem hypotheses by rigorous falsification, rising the danger of deceptive findings. Consequently, a scalable and statistically sound resolution is required to automate the speculation validation course of successfully.
Researchers from Stanford College and Harvard College launched POPPER, an agentic framework that automates the method of speculation validation by integrating rigorous statistical rules with LLM-based brokers. The framework systematically applies Karl Popper’s precept of falsification, which emphasizes disproving somewhat than proving hypotheses. POPPER employs two specialised AI-driven brokers:
The Experiment Design Agent which formulates falsification experiments
The Experiment Execution Agent which implements them
Every speculation is split into particular, testable sub-hypotheses and subjected to falsification experiments. POPPER ensures that solely well-supported hypotheses are superior by repeatedly refining the validation course of and aggregating proof. In contrast to conventional strategies, POPPER dynamically adapts its strategy based mostly on prior outcomes, considerably enhancing effectivity whereas sustaining statistical integrity.
POPPER capabilities by an iterative course of wherein falsification experiments sequentially check hypotheses. The Experiment Design Agent generates these experiments by figuring out the measurable implications of a given speculation. The Experiment Execution Agent then carries out the proposed experiments utilizing statistical strategies, simulations, and real-world knowledge assortment. Key to POPPER’s methodology is its capability to strictly management Sort-I error charges, guaranteeing that false positives are minimized. In contrast to standard approaches that deal with p-values in isolation, POPPER introduces a sequential testing framework wherein particular person p-values are transformed into e-values, a statistical measure permitting steady proof accumulation whereas sustaining error management. This adaptive strategy allows the system to refine its hypotheses dynamically, lowering the probabilities of reaching incorrect conclusions. The framework’s flexibility permits it to work with present datasets, conduct new simulations, or work together with reside knowledge sources, making it extremely versatile throughout disciplines.
POPPER was evaluated throughout six domains: biology, sociology, and economics. The system was examined in opposition to 86 validated hypotheses, with outcomes displaying Sort-I error charges under 0.10 throughout all datasets. POPPER demonstrated important enhancements in statistical energy in comparison with present validation strategies, outperforming commonplace methods resembling Fisher’s mixed check and probability ratio fashions. In a single examine specializing in organic hypotheses associated to Interleukin-2 (IL-2), POPPER’s iterative testing mechanism improved validation energy by 3.17 occasions in comparison with various strategies. Additionally, an knowledgeable analysis involving 9 PhD-level computational biologists and biostatisticians discovered that POPPER’s speculation validation accuracy was corresponding to that of human researchers however was accomplished in one-tenth the time. By leveraging its adaptive testing framework, POPPER decreased the time required for advanced speculation validation by 10, making it considerably extra scalable and environment friendly.
A number of Key Takeaways from the Analysis embrace:
POPPER offers a scalable, AI-driven resolution that automates the falsification of hypotheses, lowering guide workload and enhancing effectivity.
The framework maintains strict Sort-I error management, guaranteeing that false positives stay under 0.10, important for scientific integrity.
In comparison with human researchers, POPPER completes speculation validation 10 occasions sooner, considerably enhancing the pace of scientific discovery.
In contrast to conventional p-value testing, utilizing e-values permits accumulating experimental proof whereas dynamically refining speculation validation.
Examined throughout six scientific fields, together with biology, sociology, and economics, demonstrating broad applicability.
Evaluated by 9 PhD-level scientists, POPPER’s accuracy matched human efficiency whereas dramatically lowering time spent on validation.
Improved statistical energy by 3.17 occasions over conventional speculation validation strategies, guaranteeing extra dependable conclusions.
POPPER integrates Massive Language Fashions to dynamically generate and refine falsification experiments, making it adaptable to evolving analysis wants.
Try the Paper and GitHub Web page. All credit score for this analysis goes to the researchers of this venture. Additionally, be happy to comply with us on Twitter and don’t neglect to hitch our 75k+ ML SubReddit.
🚨 Really useful Learn- LG AI Analysis Releases NEXUS: An Superior System Integrating Agent AI System and Information Compliance Requirements to Deal with Authorized Considerations in AI Datasets
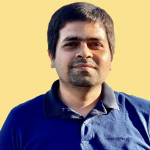
Asif Razzaq is the CEO of Marktechpost Media Inc.. As a visionary entrepreneur and engineer, Asif is dedicated to harnessing the potential of Synthetic Intelligence for social good. His most up-to-date endeavor is the launch of an Synthetic Intelligence Media Platform, Marktechpost, which stands out for its in-depth protection of machine studying and deep studying information that’s each technically sound and simply comprehensible by a large viewers. The platform boasts of over 2 million month-to-month views, illustrating its reputation amongst audiences.

[ad_2]
Source link