[ad_1]
Synthetic intelligence (AI) has made vital strides lately, particularly with the event of large-scale language fashions. These fashions, educated on large datasets like web textual content, have proven spectacular talents in knowledge-based duties corresponding to answering questions, summarizing content material, and understanding directions. Nevertheless, regardless of their success, these fashions need assistance concerning specialised domains the place knowledge is scarce or extremely particular. Coaching these fashions to carry out effectively in area of interest areas stays a major hurdle, with solely a small quantity of textual content obtainable.
A central downside in AI analysis is the inefficient means fashions purchase information from small datasets. Present fashions want publicity to hundreds of variations of the identical reality to study it successfully. This poses an issue when a reality seems solely a couple of times in a specialised corpus, making it tough for fashions to grasp and generalize from such restricted data. This inefficiency is much more pronounced when adapting a normal language mannequin to a brand new, domain-specific subject the place numerous representations of key ideas are absent.
Present AI strategies try to handle this concern by pretraining on large datasets, which provides fashions a broad understanding of normal matters. Nevertheless, this method is ineffective for domains with solely a small corpus of knowledge. Some researchers have tried to unravel this by paraphrasing the unique textual content a number of occasions to create numerous representations. Nevertheless, this technique, although simple, wants extra capacity to introduce new views or deepen understanding. After a number of rounds of rephrasing, the mannequin’s efficiency tends to plateau, as rephrasing alone doesn’t present sufficient variation for vital studying enhancements.
Researchers from Stanford College launched EntiGraph, an modern method to fixing this downside by artificial knowledge technology. The group, comprised of members from the Division of Statistics and the Division of Pc Science, developed EntiGraph to generate a big, artificial corpus from a small, domain-specific dataset. The objective is to assist fashions study extra successfully by offering a better range of examples. EntiGraph identifies key entities throughout the unique textual content after which makes use of a language mannequin to generate new, diversified content material across the relationships between these entities. This technique permits the creation of a various coaching set, even from a small quantity of information.
EntiGraph begins by extracting vital entities from a given dataset. Entities may be folks, locations, or ideas central to the textual content. After figuring out these entities, the algorithm makes use of a language mannequin to explain their relationships. These descriptions are then mixed into an artificial dataset that expands the unique corpus, offering the language mannequin with a a lot bigger and richer coaching knowledge set. This course of permits the language mannequin to study connections between entities in methods not current within the unique textual content, main to raised information acquisition. Moreover, EntiGraph organizes these relationships right into a information graph, which permits additional exploration of how totally different entities work together throughout the dataset.
The efficiency of EntiGraph was examined in a collection of experiments, and the outcomes had been promising. The researchers took a corpus of 1.3 million tokens and used EntiGraph to generate an artificial dataset containing 600 million tokens. They then pretrained a language mannequin, Llama 3 8B, on this bigger dataset. The outcomes confirmed a log-linear enchancment in accuracy because the variety of artificial tokens elevated. As an illustration, the mannequin’s accuracy in question-answering duties improved from 39.49% when utilizing the unique dataset to 56.42% after pretraining on the artificial corpus. Furthermore, the artificial pretraining utilizing EntiGraph supplied as much as 80% of the accuracy increase that fashions obtain after they can entry the unique paperwork throughout inference. This reveals that even with out entry to the unique knowledge, fashions can carry out effectively after coaching on an artificial corpus.
The examine additionally revealed that EntiGraph outperforms current strategies, corresponding to merely rephrasing the dataset. In a single comparability, the rephrased corpus contained just one.8 million tokens, and the mannequin’s accuracy plateaued at 43.08%. In distinction, EntiGraph improved mannequin efficiency even because the artificial dataset grew to 600 million tokens. The power to synthesize bigger and extra numerous datasets allowed for simpler information switch, demonstrating the prevalence of this technique in enabling language fashions to study from small, specialised datasets.
In conclusion, the introduction of EntiGraph marks a major development in addressing the challenges of information effectivity in AI fashions. The tactic efficiently generates a various, artificial corpus from a small dataset, enabling fashions to accumulate domain-specific information extra successfully. This analysis highlights a novel method that might result in additional developments in AI coaching strategies, notably for specialised fields the place knowledge is proscribed. The outcomes present that EntiGraph supplies a viable answer to overcoming the constraints of current strategies, permitting language fashions to raised adapt to area of interest domains and carry out advanced duties with improved accuracy.
Try the Paper. All credit score for this analysis goes to the researchers of this venture. Additionally, don’t overlook to observe us on Twitter and be part of our Telegram Channel and LinkedIn Group. In the event you like our work, you’ll love our publication..
Don’t Neglect to hitch our 50k+ ML SubReddit
⏩ ⏩ FREE AI WEBINAR: ‘SAM 2 for Video: Tips on how to High-quality-tune On Your Knowledge’ (Wed, Sep 25, 4:00 AM – 4:45 AM EST)
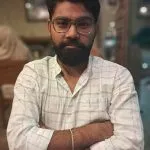
Nikhil is an intern advisor at Marktechpost. He’s pursuing an built-in twin diploma in Supplies on the Indian Institute of Expertise, Kharagpur. Nikhil is an AI/ML fanatic who’s all the time researching functions in fields like biomaterials and biomedical science. With a powerful background in Materials Science, he’s exploring new developments and creating alternatives to contribute.
[ad_2]
Source link