Multimodal embeddings mix visible and textual information right into a single representational house, enabling programs to know and relate photos and language meaningfully. These embeddings help numerous duties, together with visible query answering, retrieval, classification, and grounding. The expertise is very vital for AI fashions that interpret real-world content material via visible and linguistic lenses, resembling doc evaluation, digital assistants, or visible search engines like google and yahoo.
A urgent problem has been the lack of present fashions to generalize throughout various duties and modalities successfully. Most fashions are skilled for extremely particular duties or underperform when utilized to unfamiliar datasets. Moreover, with no broad and unified benchmark, evaluating efficiency throughout multimodal duties turns into inconsistent and fragmented. This limits the fashions’ functionality to deal with the number of capabilities required in real looking, cross-domain purposes, particularly when new information distributions are launched.
A number of instruments, resembling CLIP, BLIP, and SigLIP, have been proposed for producing visual-textual embeddings. These fashions usually use separate encoders for photos and textual content, merging their outputs via easy operations like score-level fusion. Whereas these approaches provide baseline utility, they undergo from restricted cross-modal reasoning and generalization means. Their efficiency in zero-shot circumstances tends to say no as a consequence of shallow fusion methods and the dearth of task-specific instruction dealing with throughout coaching.
In a collaboration between researchers from Salesforce Analysis and the College of Waterloo, a brand new mannequin referred to as VLM2VEC was launched alongside a complete benchmark named MMEB. MMEB contains 36 datasets throughout 4 main duties: classification, visible query answering, retrieval, and visible grounding. It divides datasets into 20 used for coaching and 16 for analysis, together with out-of-distribution duties. The VLM2VEC framework is designed to transform any vision-language mannequin into an embedding mannequin utilizing contrastive coaching. It permits it to deal with any enter mixture of textual content and pictures whereas following process directions.
To construct VLM2VEC, the analysis group used spine fashions resembling Phi-3.5-V and LLaVA-1.6. The tactic begins by setting up task-specific instruction-based queries and targets, processed via a vision-language mannequin to generate embeddings. Contrastive coaching is employed utilizing the InfoNCE loss perform with cosine similarity, aligning embeddings by maximizing the similarity between matching query-target pairs whereas minimizing it for mismatches. To help giant batch sizes, vital for coaching with various negatives, the researchers used GradCache, which splits batches into memory-manageable sub-batches and accumulates gradients. This course of ensures environment friendly coaching even with the excessive reminiscence calls for of multimodal inputs. Process-specific directions are embedded inside the coaching pipeline to assist the mannequin adapt its encoding to the character of the duty, resembling grounding or retrieval, additional boosting its generalization capabilities.
Efficiency outcomes display the benefit of the proposed methodology. The most effective-performing model of VLM2VEC used LLaVA-1.6 as its spine, utilized LoRA tuning, and processed photos at 1344 × 1344 decision. This configuration achieved a Precision@1 rating of 62.9% throughout all 36 MMEB datasets. In zero-shot checks on the 16 out-of-distribution datasets, it maintained a powerful 57.1% rating. In comparison with the best-performing baseline mannequin with out fine-tuning, which scored 44.7%, VLM2VEC confirmed an 18.2-point enchancment. In comparison with the highest fine-tuned baseline at 47.2%, the advance was 15.7 factors. Throughout all process classes—classification, VQA, retrieval, and grounding—the mannequin constantly scored above 50%, a degree of efficiency not matched by any baseline. The outcomes additionally point out that LoRA-tuned variants outperformed these skilled with full fine-tuning, exhibiting that parameter-efficient coaching methods can ship larger accuracy.
The analysis clearly outlines an answer to the issue of task-specific multimodal embedding instruments that lack generalization. By combining a well-structured coaching framework and a strong benchmark, the research demonstrates a common embedding mannequin that handles various duties successfully utilizing contrastive coaching and instruction-following. This improvement marks a significant step ahead in scalable, adaptable multimodal AI.
Take a look at Paper and Mission. All credit score for this analysis goes to the researchers of this challenge. Additionally, be happy to comply with us on Twitter and don’t overlook to affix our 85k+ ML SubReddit.
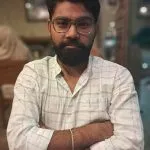
Nikhil is an intern guide at Marktechpost. He’s pursuing an built-in twin diploma in Supplies on the Indian Institute of Expertise, Kharagpur. Nikhil is an AI/ML fanatic who’s all the time researching purposes in fields like biomaterials and biomedical science. With a powerful background in Materials Science, he’s exploring new developments and creating alternatives to contribute.
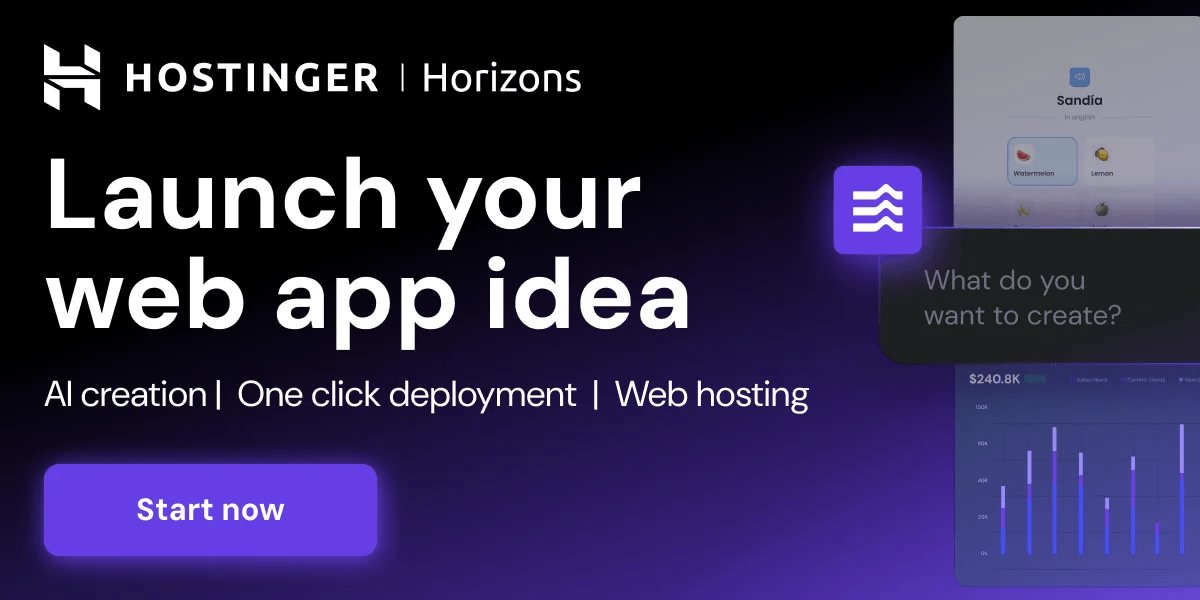