[ad_1]
Diffusion processes have emerged as promising approaches for sampling from advanced distributions however face important challenges when coping with multimodal targets. Conventional strategies based mostly on overdamped Langevin dynamics typically exhibit sluggish convergence charges when navigating between totally different modes of a distribution. Whereas underdamped Langevin dynamics have proven empirical enhancements by introducing an extra momentum variable, basic limitations stay. The degenerate noise construction in underdamped fashions the place Brownian movement {couples} not directly to the area variable creates smoother paths however complicates theoretical evaluation.
Present strategies like Annealed Significance Sampling (AIS) bridge prior and goal distributions utilizing transition kernels, whereas Unadjusted Langevin Annealing (ULA) implements uncorrected overdamped Langevin dynamics inside this framework. Monte Carlo Diffusion (MCD) optimizes targets to reduce marginal chance variance, whereas Managed Monte Carlo Diffusion (CMCD) and Sequential Managed Langevin Diffusion (SCLD) concentrate on kernel optimization with resampling methods. Different approaches prescribe backward transition kernels, together with the Path Integral Sampler (PIS), the Time-Reversed Diffusion Sampler (DIS), and the Denoising Diffusion Sampler (DDS). Some strategies, just like the Diffusion Bridge Sampler (DBS), be taught each ahead and backward kernels independently.
Researchers from the Karlsruhe Institute of Know-how, NVIDIA, Zuse Institute Berlin, dida Datenschmiede GmbH, and FZI Analysis Middle for Info Know-how have proposed a generalized framework for studying diffusion bridges that transport prior distributions to focus on distributions. This strategy incorporates each present diffusion fashions and underdamped variations with degenerate diffusion matrices the place noise impacts solely particular dimensions. The framework establishes a rigorous theoretical basis, displaying that score-matching in underdamped instances is equal to maximizing a chance decrease certain. This strategy addresses the problem of sampling from unnormalized densities when direct samples from the goal distribution are unavailable.
The framework allows a comparative evaluation between 5 key diffusion-based sampling strategies: ULA, MCD, CMCD, DIS, and DBS. The underdamped variants of DIS and DBS characterize novel contributions to the sector. The analysis methodology makes use of a various testbed together with seven real-world benchmarks masking Bayesian inference duties (Credit score, Most cancers, Ionosphere, Sonar), parameter inference issues (Seeds, Brownian), and high-dimensional sampling with Log Gaussian Cox course of (LGCP) having 1600 dimensions. Furthermore, artificial benchmarks embrace the difficult Funnel distribution characterised by areas of vastly totally different focus ranges, offering a rigorous take a look at for sampling strategies throughout various dimensionality and complexity profiles.
The outcomes present that underdamped Langevin dynamics constantly outperform overdamped alternate options throughout real-world and artificial benchmarks. The underdamped DBS surpasses competing strategies even when utilizing as few as 8 discretization steps. This effectivity interprets to important computational financial savings whereas sustaining superior sampling high quality. Concerning numerical integration schemes, specialised integrators present marked enhancements over classical Euler strategies for underdamped dynamics. The OBAB and BAOAB schemes ship substantial efficiency good points with out further computational overhead, whereas the OBABO scheme achieves one of the best total outcomes regardless of requiring double analysis of management parameters per discretization step.
In conclusion, this work establishes a complete framework for diffusion bridges that comprise degenerate stochastic processes. The underdamped diffusion bridge sampler achieves state-of-the-art outcomes throughout a number of sampling duties with minimal hyperparameter tuning and few discretization steps. Thorough ablation research verify that the efficiency enhancements stem from the synergistic mixture of underdamped dynamics, revolutionary numerical integrators, simultaneous studying of ahead and backward processes, and end-to-end realized hyperparameters. Future instructions embrace benchmarking underdamped diffusion bridges for generative modeling functions utilizing the proof decrease certain (ELBO) derived in Lemma 2.4.
Take a look at Paper. All credit score for this analysis goes to the researchers of this challenge. Additionally, be at liberty to observe us on Twitter and don’t overlook to affix our 85k+ ML SubReddit.
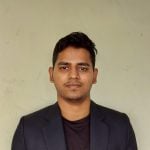
Sajjad Ansari is a ultimate 12 months undergraduate from IIT Kharagpur. As a Tech fanatic, he delves into the sensible functions of AI with a concentrate on understanding the affect of AI applied sciences and their real-world implications. He goals to articulate advanced AI ideas in a transparent and accessible method.
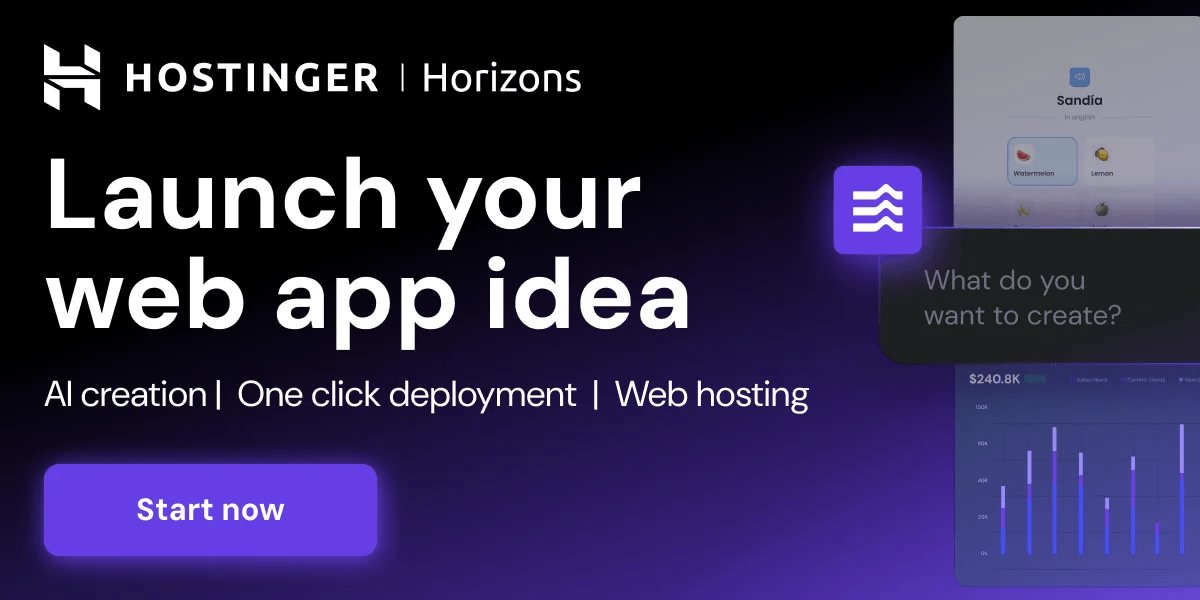
[ad_2]
Source link